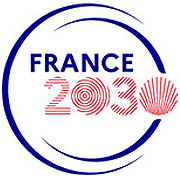
Solutions méthodologiques et logicielles pour l'intégration des données de neuro-imagerie et de génomique
Brainomics
Keywords: Neuroimaging, genetics, biomarkers, machine learning, big data
Context - Large imaging genetic studies are becoming increasingly common in research on brain diseases. In order to fully explore the collected information, analytical strategies that allow comprehensive investigations of the genetic and neural underpinnings of disorders are needed.
Objectives - The BrainOmics project aimed to implement “Big Data” tools for exploring large imaging genetic datasets. This involved: (1: WP2) the development of a software framework to manage and process complex and large data; (2: WP3) the development of new statistical methods to extract the relevant information; (3: WP4) the application of this software prototype on brain diseases.
Results - The first project’s outcome is a software prototype which targets both data management and data processing. We released three software libraries:
1. CubicWeb-DB is the database library that provides a generic framework to host, index, and query imaging-genetics data.
2. MULM-GPU is the Mass Univariate Linear Model (MULM) analysis library developed to be executed on Graphics Processing Unit (GPU). It aims to perform large scale exploration of imaging and genetic information in order to identify significant associations.
3. EPAC-CPU: Is a machine learning workflow builder designed to execute complex (non trivially portable to GPU) algorithms on CPU.
The second project’s outcome is the development of pioneering multivariate machine learning algorithms to predict some clinical outcomes based on imaging genetics information. Those mathematical developments have been implemented through two libraries:
1. ParsimonY is the multivariate (multiple input) machine learning library that enables to model biological priors (a.k.a.: structured penalties) about combined neuroimaging/genetic input data in order to predict a (single) clinical outcome.
2. Multiblock: Is the multivariate machine learning library to investigate associations between multiple input (genetic) and multiple output (imaging or clinical).
The third result consists of applications on clinical datasets of those new algorithms powered by our software prototype. On brain tumours, we used multiblock methods to identify predictive genetic markers of the tumours locations in the brain. We used the MULM-GPU method to explore brainwide - genome-wide univariate associations. We identified that the SLC39A8 (ZIP8, metal ions transporter) gene modulates the grey matter volume within the Putamen. On an in-house cohort of patients with pharmaco-resistant depression, we demonstrated that ParsimonY can perform a good prognosis of Transcranial Magnetic Stimulation (TMS) treatment response of patients using a localized atrophy within the Hippocampus. We applied ParsimonY, to the IMAGEN cohort, to predict
sub-thresholded symptoms of depression. We also used ParsimonY on neurological diseases: (i) to predict conversion to Alzheimer Disease using structural MRI, (ii) to identify the spatial patterns of white matter hyperintensities that differentially correlate with clinical severity in CADASIL disease.
The author of this summary is the project coordinator, who is responsible for the content of this summary. The ANR declines any responsibility as for its contents.
General informations
Acronym: Brainomics
Reference Number: 10-BINF-0004
Project Region: Île-de-France
Discipline: 5 - Bio Med
PIA investment: 845,021 €
Start date: November 2011
End date: November 2014
Project coordination : Vincent FROUIN
Email: vincent.frouin@cea.fr
Consortium du projet
Etablissement coordinateur : CEA Saclay
Partenaire(s) : Ecole Supérieure d'Electricité, INSERM Délégation Paris 5, Institut Gustave Roussy, LOGILAB, Alliance Services Plus, Keosys